Artificial Intelligence (AI) and data are two buzzwords that have taken the world by storm, revolutionizing industries, economies, and our daily lives. But does AI truly add value to data? Can this high-tech marriage transform mere numbers into meaningful insights? Let’s embark on a journey to uncover the relationship between AI and data, sprinkled with humor, real-world applications, and a dash of controversy to keep things interesting.
Defining AI and Data
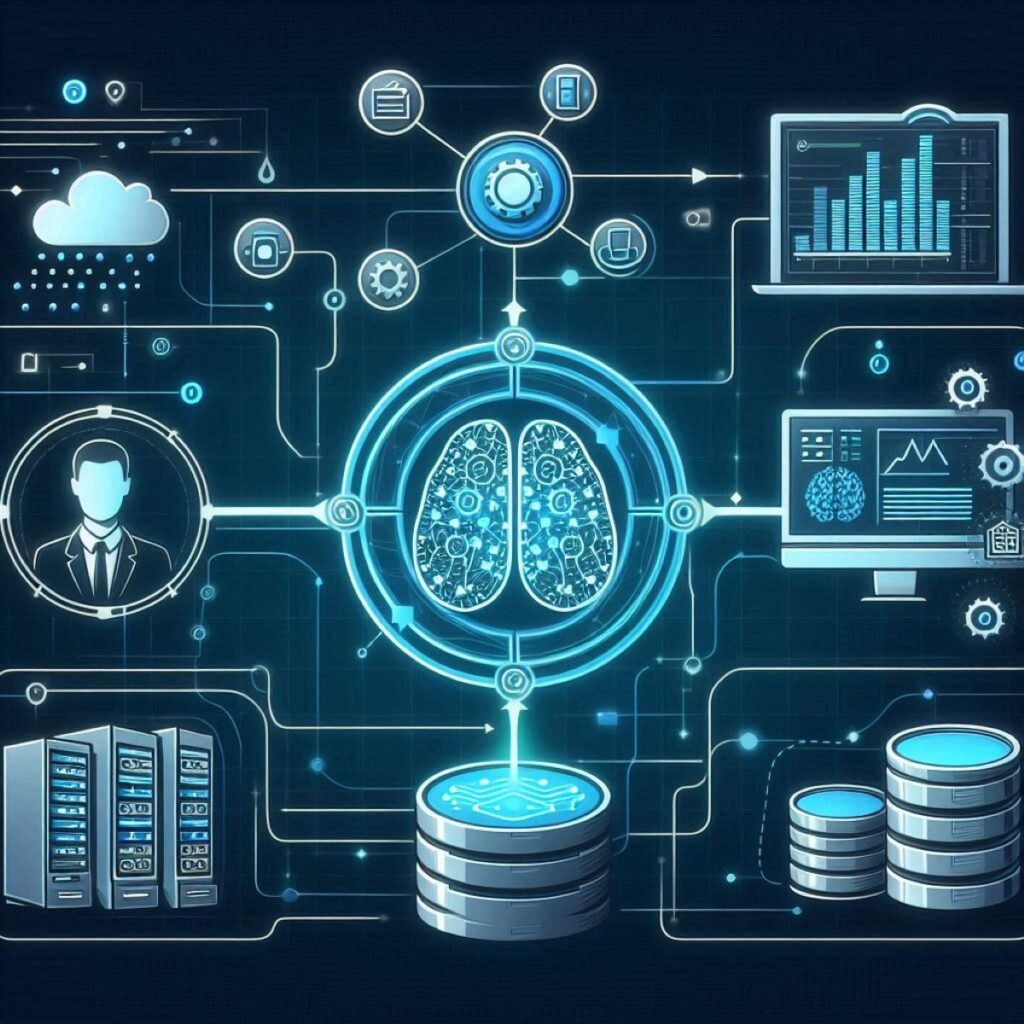
First things first, let’s break down our protagonists: AI and data. AI, in simple terms, refers to machines and systems that mimic human intelligence. These systems can learn, reason, and make decisions—think of them as super-smart interns who never ask for coffee breaks. Data, on the other hand, is raw information in the form of numbers, text, images, and more. It’s like the ingredients in a kitchen—without a skilled chef (AI), it’s just a pile of potential.
Data Processing and Analysis: The Magic of AI
Imagine you have a mountain of data—let’s say it’s all the receipts from your favorite coffee shop for the past ten years. Now, try to find a pattern in the types of coffee sold in winter versus summer. That’s a daunting task for any human, but for AI, it’s a piece of cake (or should we say, a cup of coffee). AI can sift through vast amounts of data at lightning speed, identifying patterns and insights that would take humans months or even years to uncover.
For instance, AI-driven tools like natural language processing (NLP) can analyze customer reviews to identify common themes, sentiments, and areas for improvement. This is like having an army of reviewers at your disposal, working 24/7 without ever needing a caffeine boost.
Predictive Analytics: Crystal Ball of the 21st Century
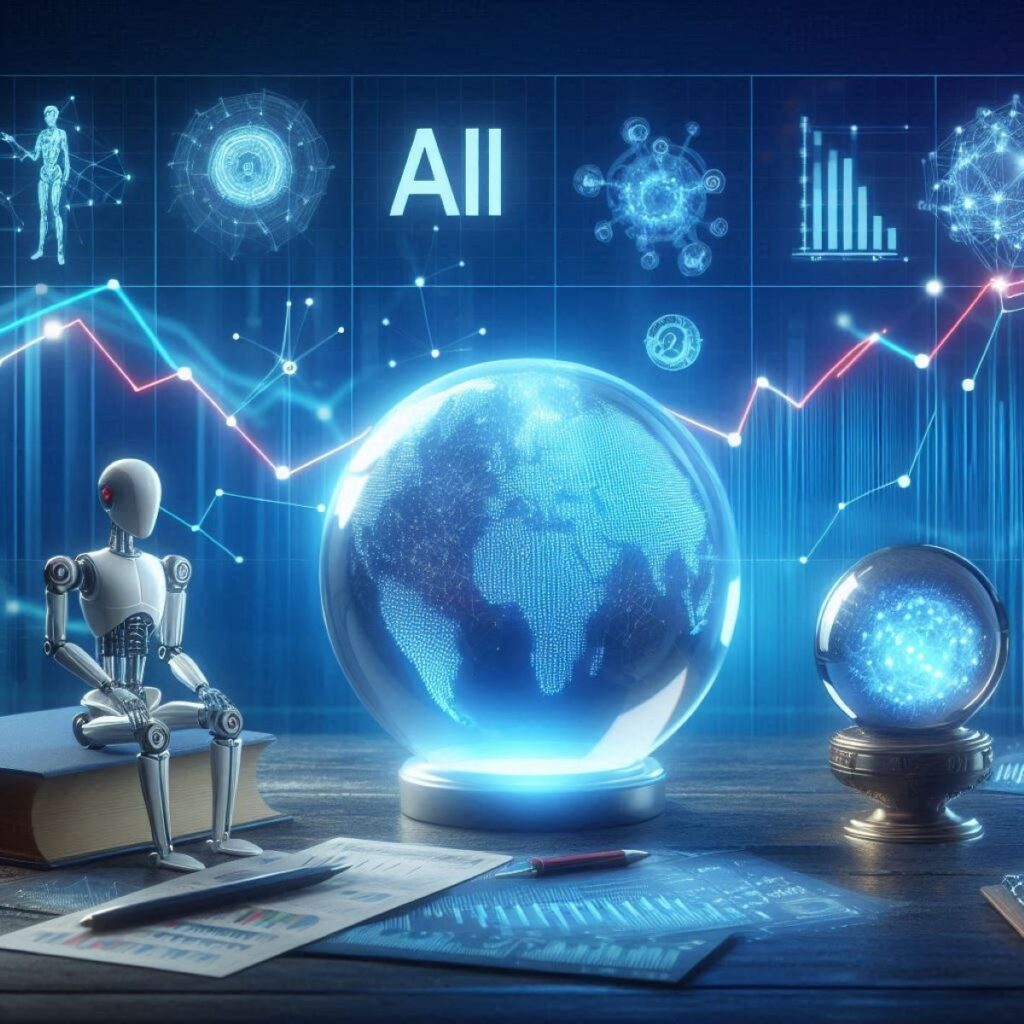
Predictive analytics is one of the most fascinating applications of AI. By analyzing historical data, AI can predict future trends and behaviors with remarkable accuracy. It’s like having a crystal ball, but instead of mystical powers, it’s powered by algorithms and data points.
Take the finance industry, for example. AI models can predict stock market trends, helping investors make informed decisions. While it might not guarantee you’ll become the next Warren Buffett, it certainly increases your chances of making profitable investments. In healthcare, predictive analytics can forecast disease outbreaks, allowing for timely interventions and potentially saving lives.
Personalization: AI’s Special Touch
One of the most compelling ways AI adds value to data is through personalization. Whether it’s recommending your next Netflix binge-watch or curating a Spotify playlist that seems to read your mind, AI uses data to create tailored experiences.
E-commerce is a prime example. AI analyzes your browsing history, past purchases, and even the time of day you shop to recommend products you’re likely to buy. This personalized approach not only enhances customer satisfaction but also boosts sales. It’s like having a personal shopper who knows your preferences better than you do.
Automation: Streamlining Repetitive Tasks
Let’s face it, some tasks are mind-numbingly repetitive. Data entry, sorting emails, and scheduling meetings can drain anyone’s energy and productivity. Enter AI-powered automation, which handles these mundane tasks with ease, freeing up humans to focus on more creative and strategic activities.
In customer service, chatbots are a perfect example of AI automation. They can handle basic inquiries, book appointments, and even troubleshoot common issues, providing instant responses and improving customer satisfaction. It’s like having a tireless assistant who never needs a day off.
Real-World Applications: AI in Action
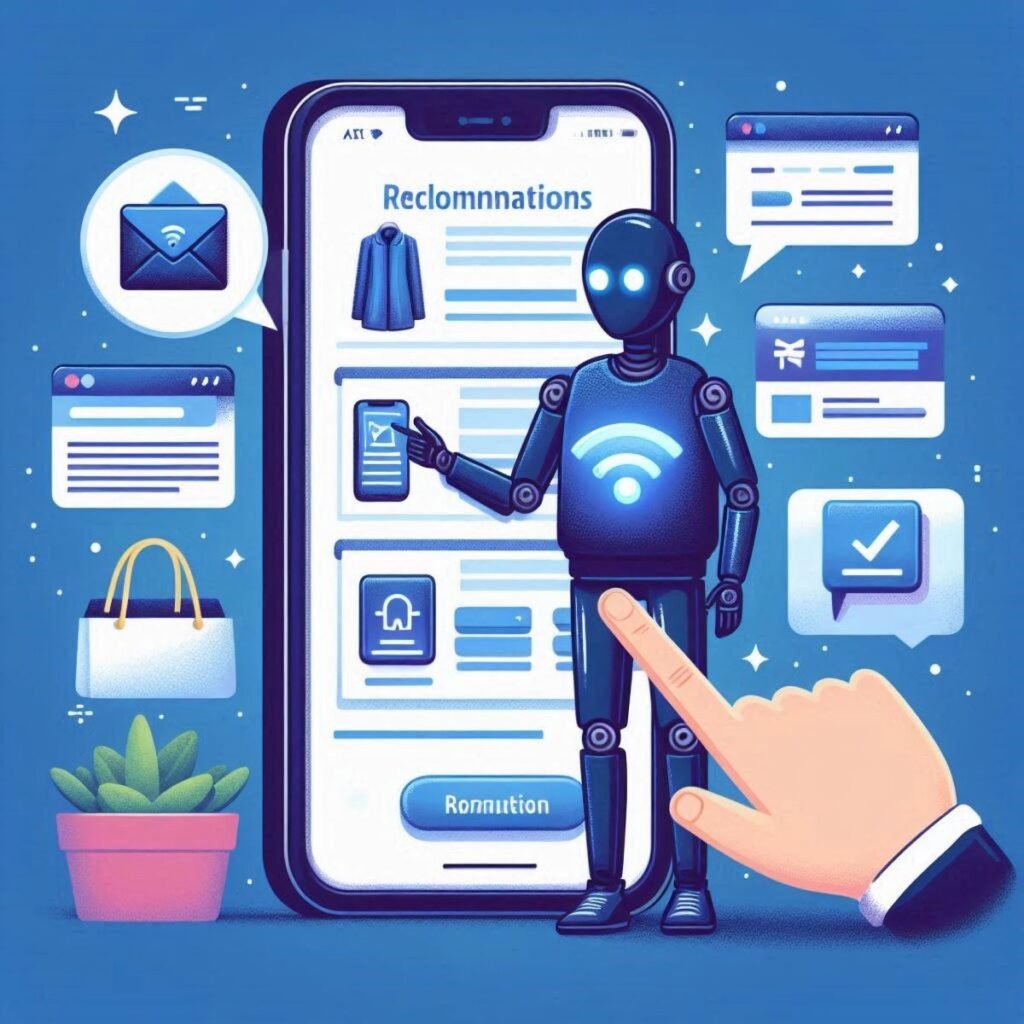
Now that we’ve explored the theoretical benefits, let’s dive into some real-world applications of AI adding value to data across various industries.
Healthcare: AI is transforming healthcare by analyzing patient data to provide personalized treatment plans, predict disease outbreaks, and even assist in surgeries. For example, IBM’s Watson Health uses AI to analyze medical records and recommend treatments, significantly reducing the time needed for diagnosis and planning.
Finance: AI helps in fraud detection, risk management, and algorithmic trading. Financial institutions use AI to analyze transaction data, detect unusual patterns, and prevent fraudulent activities. Additionally, AI-powered robo-advisors provide personalized investment advice based on individual risk profiles and financial goals.
Marketing: AI enables targeted marketing campaigns by analyzing consumer behavior and preferences. Companies like Amazon and Netflix use AI to recommend products and content, driving engagement and sales. Predictive analytics helps marketers understand which campaigns are likely to succeed, optimizing advertising spend.
Transportation: Self-driving cars, powered by AI, analyze data from sensors and cameras to navigate roads safely. Companies like Tesla and Waymo are at the forefront of this technology, aiming to reduce accidents and improve transportation efficiency.
Education: AI personalizes learning experiences by analyzing student performance data. Platforms like Khan Academy and Coursera use AI to recommend courses and provide personalized feedback, helping students learn more effectively.
Challenges and Considerations: The Flip Side of AI
While AI adds tremendous value to data, it’s not without its challenges. It’s essential to address these to ensure ethical and effective AI deployment.
Bias in AI: AI systems are only as good as the data they’re trained on. If the training data is biased, the AI will produce biased results. For example, facial recognition systems have been criticized for their inaccuracies with people of color. It’s crucial to ensure diverse and representative data sets to mitigate bias.
Privacy Concerns: The use of AI involves handling vast amounts of personal data, raising privacy concerns. Companies must implement robust data protection measures and comply with regulations like GDPR to safeguard user information.
Job Displacement: Automation through AI can lead to job displacement, particularly in industries with repetitive tasks. It’s essential to focus on reskilling and upskilling the workforce to adapt to the changing job landscape.
Ethical Considerations: The deployment of AI should align with ethical guidelines to prevent misuse. For instance, using AI for surveillance or manipulation raises ethical questions that need to be addressed through regulation and oversight.
Conclusion: Balancing Potential and Challenges
So, does AI add value to data? The answer is a resounding yes. From processing and analyzing data at unprecedented speeds to providing personalized experiences and automating mundane tasks, AI significantly enhances the value derived from data. However, it’s crucial to balance this potential with considerations of bias, privacy, job displacement, and ethical use.
As we navigate the AI-driven future, it’s important to continue exploring and addressing these challenges. By doing so, we can harness the power of AI to unlock new opportunities, drive innovation, and create a more equitable and efficient world.
In the end, AI’s value to data is not just about the technology itself but how we choose to use it. With thoughtful implementation, AI can indeed transform data from a pile of potential into a treasure trove of insights and solutions.
As you ponder these ideas, consider this: In a world where data is the new oil, AI is the refinery that turns it into fuel for progress. Keep coming back for more insights, and let’s continue this journey together, exploring the ever-evolving landscape of AI and data.